Introducing LightlyEdge: Smarter Data Collection, Lower Costs for Edge AI
We are excited to announce LightlyEdge (LightlyEdge) – a smart data collection SDK built for edge AI devices. In an era of global competition in ADAS, video, and robotics, companies face data overload from millions of driving miles and sensor hours that is being collected by their fleets. This rise of data volumes leads to teams being overwhelmed with what to store, label, and train AI from. Uploading everything to the cloud is impractical, costly, and inefficient. Critical corner cases often hide in a sea of unstructured footage, and teams struggle to find the needles in the haystack.
LightlyEdge changes this by making your devices smarter - capturing only data that matters, right at the source in real-time at the edge, drastically cutting down the data flood into actionable insights.
Why launch LightlyEdge now?
The autonomous driving and edge AI industry is at an inflection point. Vehicles and devices are more powerful than ever (thanks to chips like NVIDIA Orin), yet data pipelines haven’t caught up. Developers are still forced to either log everything (burying themselves in data) or rely on simplistic triggers that miss important events. Meanwhile, privacy regulations and cloud/bandwidth costs discourage bulk data uploads. LightlyEdge arrives at the perfect time – it transforms edge devices into intelligent gatekeepers that decide on the fly which data is worth keeping. By deploying LightlyEdge, organizations can stay ahead of global competition with faster iteration on real-world scenarios, all while controlling costs and complying with data locality requirements.

Smart Data Capture: Bringing Observability to the Edge
Edge AI observability means having eyes on what your models and sensors are seeing in the field – and acting on it. Until now, teams lacked a comprehensive way to do this on-device. We built one of the most widely used open-source tools for data selection and model training in ML, and we’ve spent years working with automotive clients on their data challenges. We heard the same pain points:
“Our R&D cars generate petabytes of video – we are collecting too much of the same data and we can’t afford to save it all, but we’re terrified of missing important edge cases.”
There was a clear need for a solution that operates where the data is collected. So, we set out to make edge observability and smart data capture easy. LightlyEdge is the result of many iterations with our partners – an SDK that distills powerful machine learning techniques into a lightweight package for the edge. It runs directly on vehicles, robots, or cameras, continuously enriching each frame with AI insights and deciding in real-time whether to keep it (LightlyEdge Python SDK — LightlyEdge Python Bindings documentation). By filtering data at the source, LightlyEdge bridges the gap between raw sensor input and model training data.
The end goal: Ensure you capture just the data you need – those rare events, edge cases, and new scenarios – without overwhelming your storage or labeling pipeline.
Key features and workflows
LightlyEdge helps teams unlock the value of their domain-specific data right at the point of capture. By automatically selecting only the most informative, high-signal frames from a video stream, it significantly reduces the volume of data you need to transfer and store. This cuts costs on cloud storage and manual review, and speeds up model improvement cycles. Instead of sifting through terabytes of redundant footage, engineers can focus on new features and hard problems. LightlyEdge integrates seamlessly into existing camera or sensor setups and works out-of-the-box with popular automotive hardware. It’s available as a simple SDK (in Python or C++), running fully on-device with no internet connection required for complete data privacy.
Key features and benefits:
- 🧩Gap-Filling Diversity: LightlyEdge ensures your dataset covers the long-tail scenarios. It uses diversity selection to pick out frames that are visually distinct from ones you’ve already saved to storage. This way, if your fleet encounters a new weather condition or an unusual environment, those frames get captured. By filling in distribution gaps, you build more robust models that aren’t blind to rare conditions.
- 🔍Edge-Case Mining: The SDK automatically flags and stores rare or novel occurrences in your data. It can detect when a frame looks fundamentally different (out-of-distribution) or when an unusual object or scenario appears (for example, a new construction zone on the road). These edge cases are often the incidents that cause model failures. LightlyEdge acts like an always-on treasure hunter for such critical data, including the ability to detect novel scenarios and domain shifts in real-time so you can retrain your models before they falter.
- 🧠Vision-Language Search: Finding specific scenarios in hours of video used to be like finding a needle in a haystack. LightlyEdge changes that by bringing vision-language search to the edge. Powered by advanced vision-language embeddings, it lets you query your footage with text or images. Simply provide a text description (e.g. "ambulance with siren" or "snowy road at night") or a reference image, and LightlyEdge will highlight frames that match. This foundation-model capability runs on-device, so you can identify events of interest without deploying new specialized models for each query.
- 🎯Enrichment Triggers: Every frame that passes through LightlyEdge is first enriched with semantic information by lightweight neural networks. This includes metadata like embeddings, object detections, classifications from vision-language search, and more. You can then set custom triggers based on these enrichments and CANbus data (e.g., velocity, blinker flashing). For example, you might configure: “Save the frame if it contains a pedestrian at 60 mph” or “Capture any frame that is at least 30% different (in embedding space) from what I’ve seen so far.” These triggers are highly configurable using our SDK, enabling automation of complex data collection policies. The result is an optimized dataset growth – you collect different and meaningful data, not more of the same.
- 📊Edge Analytics & Live Monitoring: LightlyEdge doesn’t just select data, it also provides visibility into what your devices see. The SDK includes monitoring capabilities that display the frequency and distribution of events or objects your edge device encounters. Imagine knowing in real-time how often your delivery robots see crosswalks or how many times your drone spotted a certain animal. This live analytics view gives you unprecedented insight into your fleet’s operating environment. It’s a dashboard of your data’s diversity and gaps, enabling data-driven decisions on the fly. In short, LightlyEdge makes your edge data measurable and actionable, closing the loop for continuous learning.
How does it work
Getting started with LightlyEdge is simple — yet powerful. The SDK follows a 3-step process to turn edge devices into intelligent, real-time data selectors:
1️⃣ Configure Smart Triggers

Define what you’re looking for — whether that’s:
- Specific object classes (e.g. bicycles, stop signs, snowplows)
- Vision-language prompts (e.g. “pedestrian in crosswalk at night”)
- Trigger thresholds for rarity, diversity, or embedding distance
These criteria can be tailored to your project goals — closing dataset gaps, collecting diverse road scenarios, or spotting rare events.
2️⃣ Run On the Vehicle
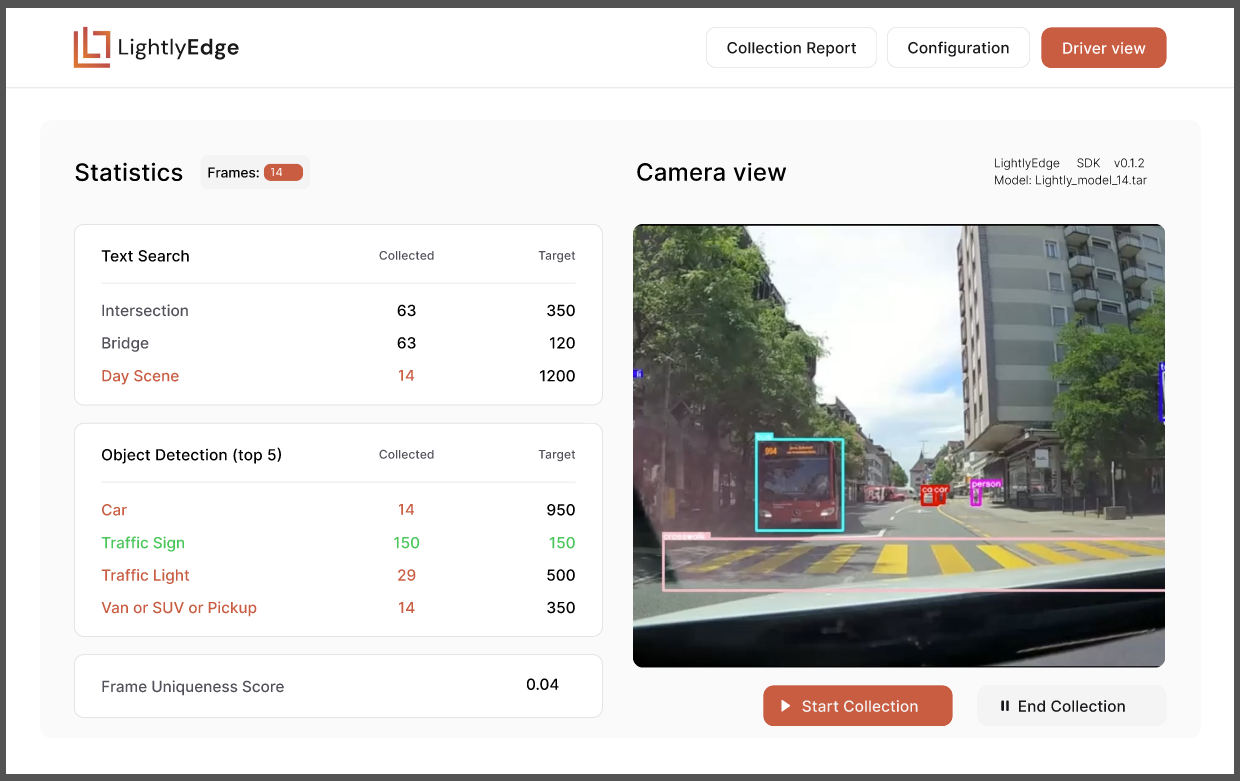
Deploy the SDK directly onto your edge device — whether it’s an in-car Jetson Orin or a roadside camera system. LightlyEdge evaluates every incoming frame in real time, using your configured rules to decide what gets captured. For instance, if your goal is to collect 200 diverse examples of snowy intersections, LightlyEdge monitors the live stream and stops saving once the quota is met.
3️⃣ Review the Collection Report

At the end of a run, you receive a collection summary that shows:
- How many frames were captured and it’s distributions
- What classes or prompts triggered captures
- How diverse or unique the final dataset is
This final report gives you observability and confidence that your dataset is both high-quality and purpose-built — without the cloud bloat.
Built for Real-Time Edge Performance
LightlyEdge was built from the ground up to run efficiently on automotive and edge hardware. We know that edge devices have limited compute and memory, so every component of LightlyEdge is optimized. Even on the modest NVIDIA Jetson Orin Nano 8GB – a common edge AI platform in vehicles – LightlyEdge achieves real-time throughput. In our benchmarks, the SDK sustains >100 frames per second when using the Orin Nano’s GPU, and >10 FPS even with CPU-only processing. This means you can deploy it on a low-power vehicle computer and still analyze video frames faster than the cameras can capture them.
Despite its capabilities, LightlyEdge remains lightweight. The entire runtime (models and code) has a footprint of under 200 MB on disk, and it can operate with as little as 128 MB of RAM. It’s a perfect fit for embedded systems. The SDK’s core is written in Rust for efficiency and safety, with carefully designed neural models that balance accuracy and speed. In practice, this means you get state-of-the-art semantic search and detection on edge devices without needing a beefy GPU or endless memory. From fanless AI cameras to automotive ECUs, if your hardware can run a basic neural network, it can run LightlyEdge – and do so at speeds that keep up with live video.
Benchmarks on Jetson Orin™ Nano 8GB
We offer Python and C++ Edge SDK. Thanks to both SDKs being written in Rust, the performance is comparable.
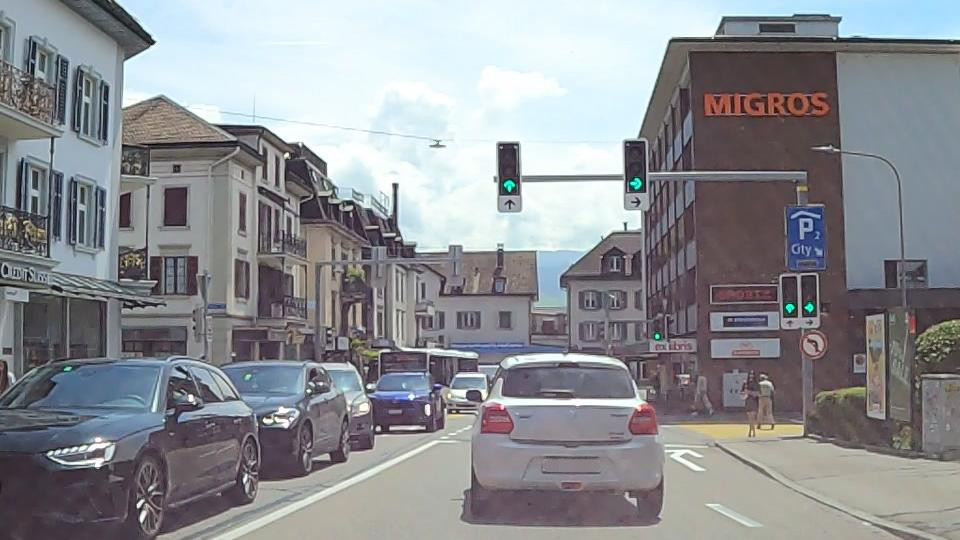
Our SDK can perform simple text search and object detection. We’ve tested the speed of both operations in Python and C++ on a NVIDIA Jetson Orin™ Nano device. Here are the results:
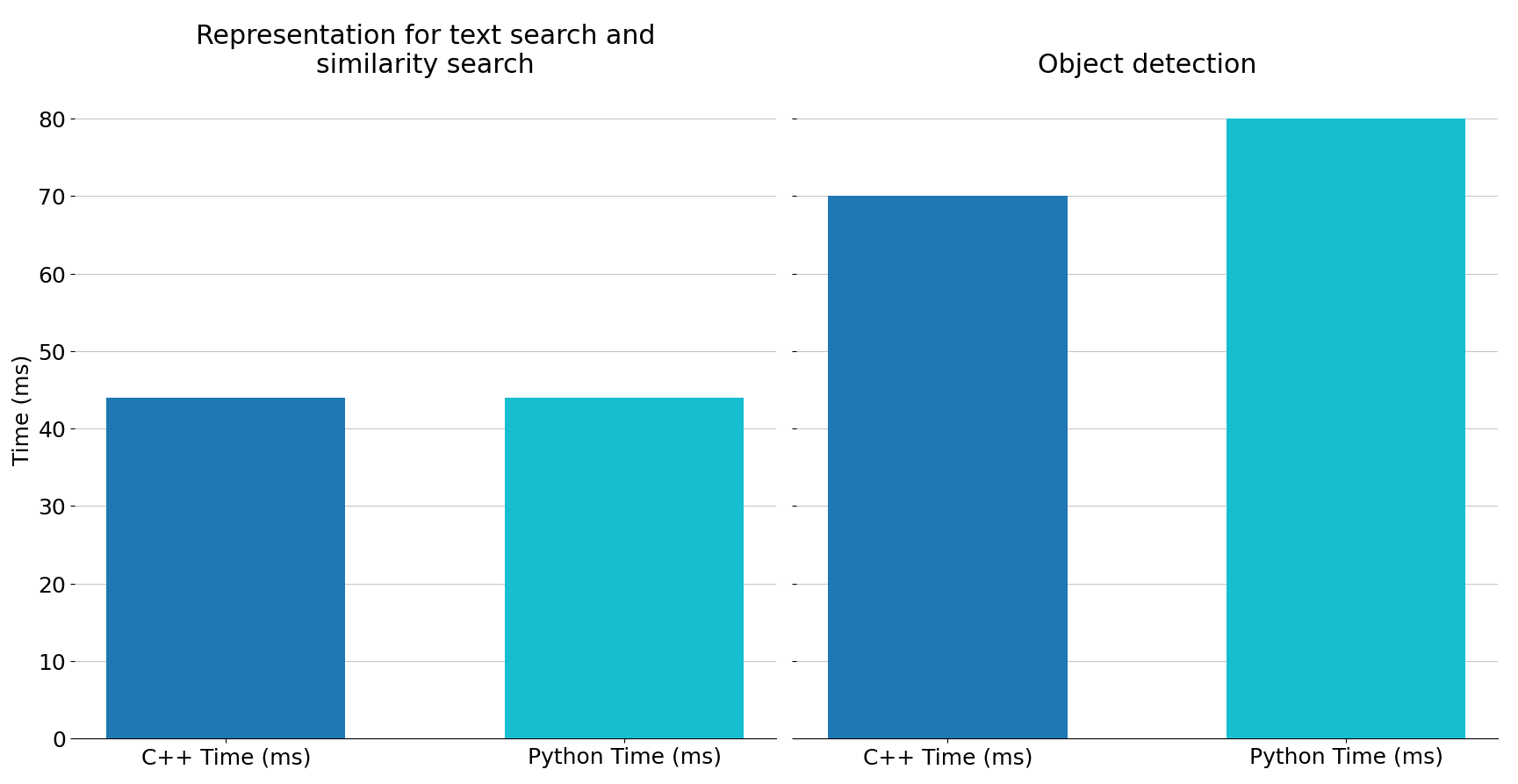

Benchmark details:
- Jetson Orin™ Nano 8GB
- Ubuntu 22 LTS with NVIDIA JetPack 6.0
- CUDA 12 and cuDNN 8
- Rust compiler 1.81, Python 3.10, gcc 11
- Image preprocessing not included
Developer-Friendly Integration

We built LightlyEdge to be as developer-friendly and flexible as possible. The SDK comes with bindings in both C++ and Python, so you can integrate it into a wide range of applications – whether it’s a native C++ autonomous driving stack or a Python-based research pipeline. Under the hood, the heavy lifting is done in highly optimized Rust code, but as a developer you interact with simple and intuitive APIs. For example, in Python you can initialize the LightlyEdge engine with one call and immediately start passing frames to it for selection; in C++, you can do the same with our provided library. We handle all the low-level details like device memory management and CUDA inference – so if there’s an NVIDIA GPU available, LightlyEdge will automatically use it to accelerate embedding and detection. If no GPU is present, it gracefully falls back to CPU processing. This automatic optimization means you write your code once and let LightlyEdge figure out the fastest way to run on the given hardware.
Compatibility is a non-issue. LightlyEdge supports all major operating systems and architectures out of the box – Linux, Windows, and MacOS, on both x86_64 and ARM platforms. Whether you’re deploying to an Ubuntu server in a data center or a custom ARM board in a drone, the SDK has you covered. And since no internet connection is required to run LightlyEdge, you can deploy it in secure or offline environments (common in automotive scenarios) without worry.
To make getting started even easier, we include pre-trained models tailored for automotive and similar visual tasks. LightlyEdge ships with in-house trained embedding models and object detectors that know how to recognize a variety of road scenes and objects (cars, pedestrians, traffic signs, etc.). This means right out-of-the-box, your edge device is equipped with domain-specific understanding. If your use-case is automotive, you’ll benefit from our highly curated training. And for other domains, LightlyEdge’s modular design lets you swap in your own models or work with us to extend support – ensuring the SDK can adapt to your particular edge AI needs.
Moving the Industry Forward
LightlyEdge represents a major step forward for the edge AI and automotive industry. By solving the edge observability problem, it enables a new paradigm: continuous learning from the fleet. No longer is valuable driving data locked away on hard drives or discarded due to volume – the fleet itself becomes a smart filter and contributor to the ML pipeline. This closes the loop between deployment and development. Engineers can iterate faster on real-world feedback, leading to safer autonomous systems and more capable AI on our roads and in our devices.
Strategically, LightlyEdge aligns with the industry’s push toward decentralized, intelligent systems. It transforms vehicles and edge devices from passive data generators into active participants in model improvement. This not only reduces the data engineering burden (and cloud costs), but also paves the way for scaling autonomous fleets without drowning in data. Teams can maintain model performance edge by continuously feeding models the right data – even as conditions and geographies change – confident that they’re not missing the next important scenario.
We believe LightlyEdge will help usher in a future where edge AI is smarter, leaner, and more autonomous. It’s a solution built on the idea that better data beats more data, and that the best place to decide what data matters is at the source. We’re thrilled to see how LightlyEdge is going to supercharge the way we develop AI models.
LightlyEdge is available now. To learn more, visit the LightlyEdge product page or check out our developer documentation for a deep dive into the SDK. We can’t wait for you to try it out and bring observability to the edge in your own projects. Here’s to a new era of intelligent data collection and better models, right at the edge!