AIGEN
Making farming smarter with better computer vision
About
Aigen, an emerging enterprise, is revolutionizing agriculture with its unique robots designed to aid farmers. These innovative machines operate without the need for detrimental chemicals or fossil fuels, relying instead on solar energy and computer vision. They effortlessly navigate farm terrains, adept at identifying weeds and inspecting crops, thereby simplifying and eco-friendly farming operations.
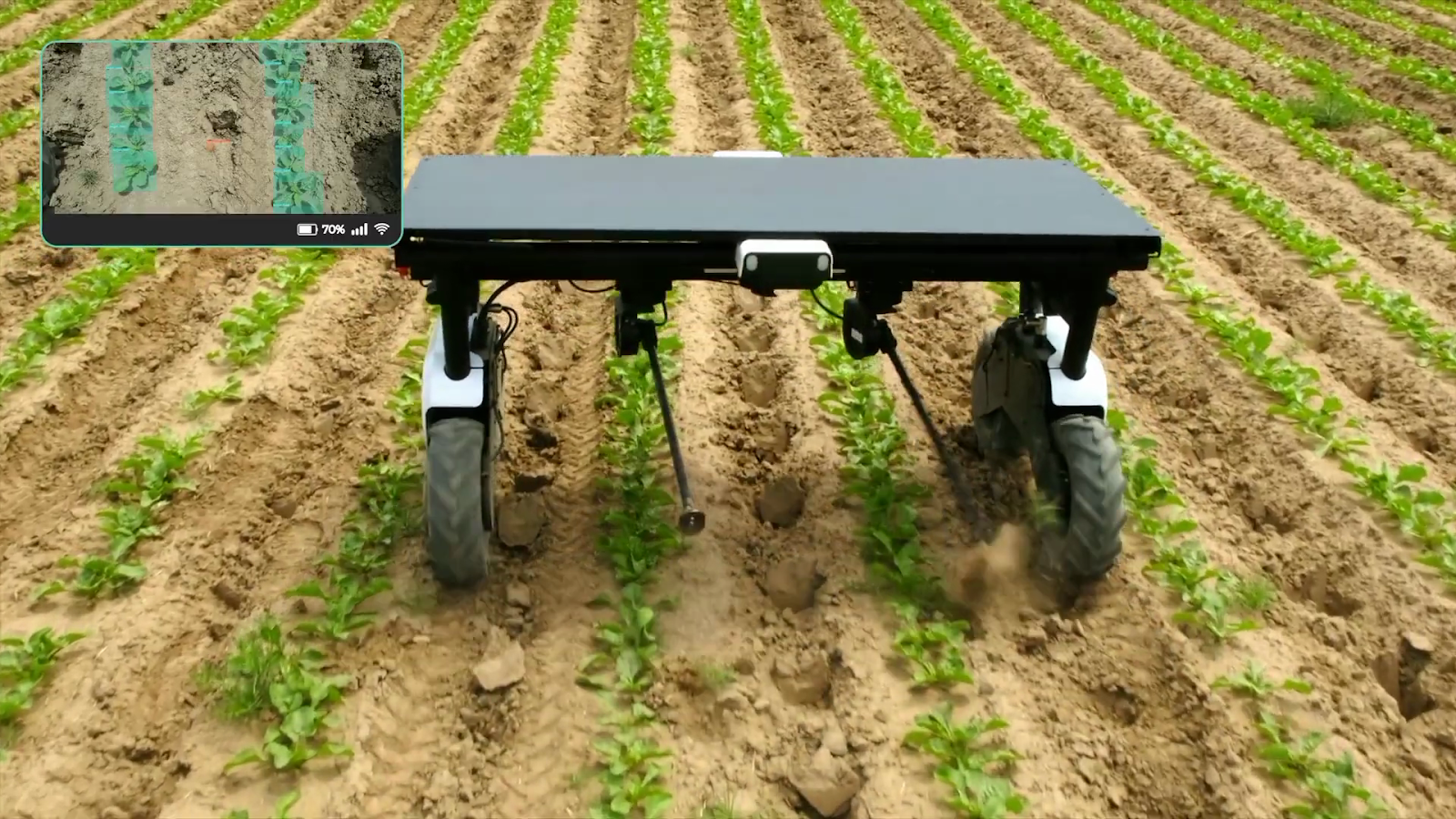
Problem
Developing a computer vision system capable of accurately recognizing and interpreting crop fields presents significant challenges. Agricultural environments are complex, characterized by varying weather, different soil types, and uneven landscapes, which complicates the robots' ability to discern and understand their surroundings. To enhance their machine learning models, Aigen faced the crucial task of acquiring and effectively utilizing the right data for training.
The main challenges for Aigen are:
- Understanding the data distribution and getting insights into their data
- Finding the relevant data to use for labeling and retraining the ML models
- Ensuring the ML models reliably work on different terrains (e.g.,weather, soil) and crops
Scalable and Efficient Data Curation using Lightly
Specializing in sorting and improving data for training robots, Lightly helped Aigen to pick edge cases. This ensured covering many different scenarios Aigen’s robots may face on the fields. It provided learning data for all sorts of different farming situations. As a results robots became smarter and performed better.
Aigen used Lightly on a pre-defined workflow that was established in their machine learning pipeline. It included the following 6 steps:
- Data collection: Recording videos and images with robots on the fields
- Data ingestion: Upload data to their S3 bucket
- Automated data curation: Running the selection configuration of Lightly to select the best subset of the collected data for further processing
- Manual data curation: Efficient fine-tuning of the data by an agronomist and data expert of Aigen in the Lightly User Interface
- Push data to labeling tool: Leverage Lightly’s integration to push data to Labelbox tool for labeling
- Train model: Train model on the new data, evaluate results and restart the process with step 1.

Results
Overall the collaboration with Lightly yielded remarkable improvements in Aigen's machine learning capabilities.
- 2x efficiency gains: The deployment process became twice as efficient
- 80% dataset size reduction: Through Lightly's smart data collection the total size of the dateset was reduced significantly saving data storage costs
These advancements mean Aigen's robots are now more effective in maintaining crop health without relying on chemicals or extensive human intervention.
Aigen achieved these improvements by employing a blend of automated and human-aided workflows. Initially, automated pipelines utilizing Lightly's data selection algorithms identified potential edge cases. Subsequently, the Lightly platform's user interface was employed for further refinement of this data before sending it to their labeling tool. This strategic approach significantly contributed to the overall efficiency and effectiveness of Aigen's technological solution in agriculture.
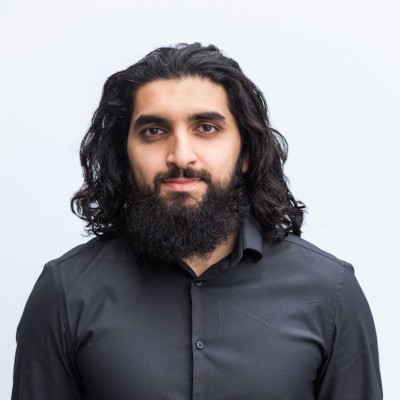
“By integrating Lightly into our existing workflow, we achieved a 90% reduction in dataset size and doubled the efficiency of our deployment process. The tool’s seamless implementation significantly enhanced our data pipeline.”
H1
H2
H3
H4
H5
H6
Paragraph
- list
- list
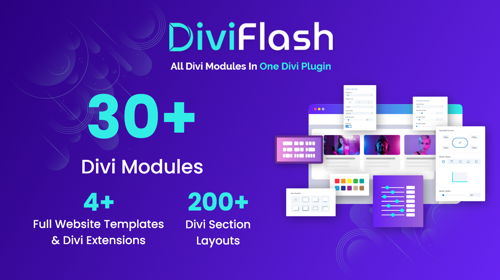